Thalictrum Scent Part 2
Written on February 25th, 2019 by Jesus Martinez-Gomez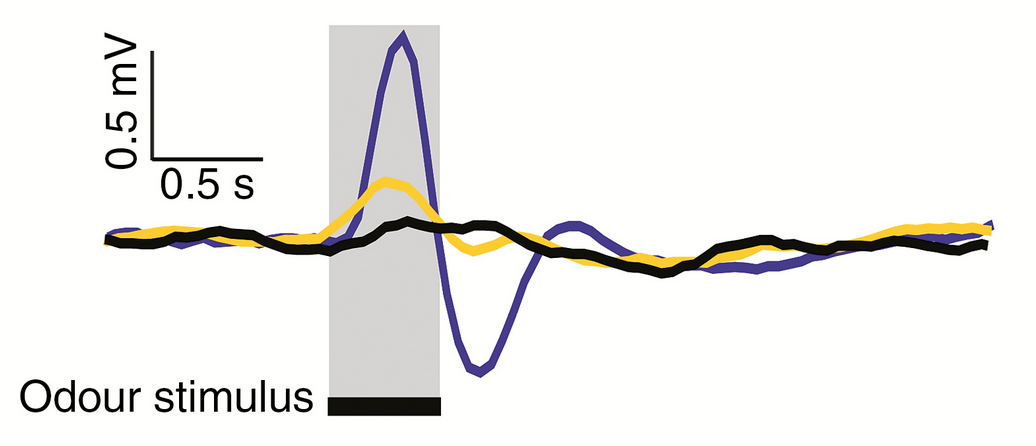
This is the second post going through the code I wrote for our floral scent paper Annuals of Botany. Check out the part 1 here including a little more introduction on the project.
Here we ask How similar/different are floral scent profiles between wind and insect pollinated species? In this script we calculate Blombergs k for our emission data and electroantennogram response a measrument of phylogentic signal (how similar closely related species).
The Data
1) Floral scent analyzed by gas chromatography-mass spectrometry from 11 species of Thalictrum.
2) Electroantennogram (EAG) responses from buff-tailed bumblebee antenna exposed to floral scent extract from 8 Thalictrum species. I think this kind of data is really cool.
Test for Phylogenetic Signal in R
Load libraries and data
library(phytools)
library(geiger)
library(picante)
library(phylocurve)
library(geomorph)
library(tidyverse)
scent <- read.csv("assets/code/scent/compounds.csv") # Import Scent GCMS floral scent data
tree <-read.nexus("assets/code/scent/THALICTRUM86.tre") # Read in pre-cooked Maximum A Posterior Phylogeny (MAP)
EAG <- read.csv("assets/code/scent/EAGresponses.csv") # Import EAG data
Test for Phylogenetic Signal
We were intrested in testing whether closely related species exhibitd similar amounts of volitale compounds. We can do this by calculating Blombergs K a measure of phylogenetic signal. Generally you can interpret K as the following
K < 1 closely related species are less similar than expected under brownian motion. K = 1 closely related species are as similar/different as expected under brownian motion K >1 closely related species are more similar than expected under brownian motion.
This is traditionally performed on single trait, however biologist are often intrested in how complex traits composed of many different variable evolve. Because our GCMS data is multivariate (~50 compounds), we can calculate Blombergs K for each individaul compound as well as the entire datset as a whole.
Prune MAP phylogeny to species with GCMS data
keepSpecies <- unique(as.character(scent$Species_Name_Full))
trimedTree <- drop.tip(tree,tree$tip.label[-match(keepSpecies, tree$tip.label)])
plot(trimedTree)
Extract scent emission data take the averge of biological replicates and wrangle the data into the proper formate for the downstream functons.
#1)
scentCompounds <- scent[,9:63] # extract only scent data + mass*time column
scentCompounds$Species_Name_Full <- scent$Species_Name_Full # Add species names back in
#2) Average Scent Data Accross Biological Replicates
avgScentCompounds <- aggregate( scentCompounds,
by=list(scentCompounds$Species_Name_Full),
FUN = mean,
na.rm=TRUE)
#3)
avgScentCompounds$Species_Name_Full <- NULL
row.names(avgScentCompounds) <- avgScentCompounds$Group.1
avgScentCompounds$Group.1 <- NULL
avgScentCompounds <- as.matrix(avgScentCompounds)
avgScentCompounds[,1:5]
Bloombergs K
Data is formated, we can now calculate Blombergs K for each compound individually.
multiPhylosignal(avgScentCompounds, tree)
## K
## Mass.time 0.7385669
## Limonene 0.3340407
## X3.Hexen.1.ol...Z.. 0.2492910
## X1.Hexanol 0.3883188
## beta.Pinene 0.2681185
## X3.Hexen.1.ol..acetate...Z.. 0.2486036
## trans.beta.Ocimene 0.3702101
## Caryophyllene 0.3215032
## Humulene 0.3890120
## alpha.Farnesene 0.3560754
## X3.Carene 0.3508240
## Methyl.Benzoate 0.3590712
## X.E..beta.Famesene 0.2374881
## Germacrene.D 0.2434557
## Pentadecane 0.7406907
## Heptadecane 0.5174426
## alpha.Pinene 0.2902098
## Camphene 0.4374292
## beta.Myrcene 0.3631219
## Tridecane 0.7857405
## Tetradecanal 0.7872098
## Benzaldehyde 0.4779120
## Eucalyptol 0.6550058
## X1.Dodecene 0.6366995
## Tetradecanoic.acid..ethyl.ester 0.6550058
## X1.Octanol 0.4106495
## Octanoic.acid..ethyl.ester 0.2619039
## Dodecane 0.5891558
## Tetradecane 0.6939679
## Hexadecane 0.8954568
## Hexadecanoic.acid..ethyl.ester 0.6550058
## Anisole 0.2576813
## Acetophenone 0.4970347
## Benzyl.Benzoate 0.5489103
## Butanedioic.acid..diethyl.ester 0.4970347
## Benzeneacetic.acid..ethyl.ester 0.4970347
## Undecane 0.7975390
## Decanal 0.7891057
## Dodecanal 0.7981688
## Hexanal 0.2460810
## X2.Hexenal 0.2412507
## Decane 0.2412507
## Butanoic.acid..3.hexenyl.ester...Z.. 0.2412507
## Styrene 0.2676727
## X2.6.Octadien.1.ol..3.7.dimethyl....Z.. 0.2676727
## X2.6.Octadienal..3.7.dimethyl....Z.. 0.2676727
## X2.6.Octadien.1.ol..3.7.dimethyl....E.. 0.2676727
## X2.6.Octadienal..3.7.dimethyl. 0.2676727
## X2.Propen.1.ol..3.phenyl. 0.2676727
## X6.Octen.1.ol..3.7.dimethyl...acetate 0.2666540
## X2.6.Octadien.1.ol..3.7.dimethyl...acetate...Z.. 0.3545785
## Geranyl.acetate 0.4100613
## gamma.Terpinene 0.2374881
## Decanoic.acid..ethyl.ester 0.2297105
## beta.Phellandrene 0.2297105
## PIC.variance.obs
## Mass.time 1.991460e+09
## Limonene 1.454599e+15
## X3.Hexen.1.ol...Z.. 4.180165e+16
## X1.Hexanol 1.097690e+13
## beta.Pinene 2.001118e+16
## X3.Hexen.1.ol..acetate...Z.. 1.367412e+18
## trans.beta.Ocimene 4.597924e+16
## Caryophyllene 8.968954e+15
## Humulene 3.311644e+15
## alpha.Farnesene 1.500355e+16
## X3.Carene 5.576785e+15
## Methyl.Benzoate 5.850166e+14
## X.E..beta.Famesene 2.666147e+13
## Germacrene.D 1.253323e+15
## Pentadecane 6.695815e+15
## Heptadecane 4.764546e+14
## alpha.Pinene 2.191704e+16
## Camphene 1.838358e+15
## beta.Myrcene 5.514877e+13
## Tridecane 6.540483e+15
## Tetradecanal 6.654161e+14
## Benzaldehyde 1.700728e+13
## Eucalyptol 2.803620e+15
## X1.Dodecene 1.020432e+14
## Tetradecanoic.acid..ethyl.ester 1.891866e+13
## X1.Octanol 1.672309e+13
## Octanoic.acid..ethyl.ester 1.058169e+14
## Dodecane 4.019885e+12
## Tetradecane 3.711310e+13
## Hexadecane 2.711331e+13
## Hexadecanoic.acid..ethyl.ester 2.559743e+12
## Anisole 3.533787e+14
## Acetophenone 4.646764e+11
## Benzyl.Benzoate 8.638989e+12
## Butanedioic.acid..diethyl.ester 6.341013e+12
## Benzeneacetic.acid..ethyl.ester 7.689925e+13
## Undecane 1.631756e+14
## Decanal 1.051953e+14
## Dodecanal 9.874612e+14
## Hexanal 1.236383e+13
## X2.Hexenal 1.480362e+14
## Decane 7.008554e+11
## Butanoic.acid..3.hexenyl.ester...Z.. 1.866172e+12
## Styrene 2.517338e+13
## X2.6.Octadien.1.ol..3.7.dimethyl....Z.. 1.539837e+16
## X2.6.Octadienal..3.7.dimethyl....Z.. 1.618029e+14
## X2.6.Octadien.1.ol..3.7.dimethyl....E.. 3.002541e+16
## X2.6.Octadienal..3.7.dimethyl. 1.768330e+14
## X2.Propen.1.ol..3.phenyl. 3.514822e+13
## X6.Octen.1.ol..3.7.dimethyl...acetate 1.313822e+15
## X2.6.Octadien.1.ol..3.7.dimethyl...acetate...Z.. 2.423591e+13
## Geranyl.acetate 2.031495e+15
## gamma.Terpinene 8.746335e+11
## Decanoic.acid..ethyl.ester 1.371433e+12
## beta.Phellandrene 7.773881e+12
## PIC.variance.rnd.mean
## Mass.time 3.083641e+09
## Limonene 1.284458e+15
## X3.Hexen.1.ol...Z.. 2.591206e+16
## X1.Hexanol 9.771308e+12
## beta.Pinene 1.320734e+16
## X3.Hexen.1.ol..acetate...Z.. 8.399416e+17
## trans.beta.Ocimene 4.048679e+16
## Caryophyllene 6.455426e+15
## Humulene 3.017447e+15
## alpha.Farnesene 1.432536e+16
## X3.Carene 4.454861e+15
## Methyl.Benzoate 5.615591e+14
## X.E..beta.Famesene 1.584537e+13
## Germacrene.D 7.557873e+14
## Pentadecane 1.370209e+16
## Heptadecane 6.540575e+14
## alpha.Pinene 1.500086e+16
## Camphene 2.056997e+15
## beta.Myrcene 4.583779e+13
## Tridecane 1.326472e+16
## Tetradecanal 1.383503e+15
## Benzaldehyde 2.121307e+13
## Eucalyptol 5.032496e+15
## X1.Dodecene 1.768018e+14
## Tetradecanoic.acid..ethyl.ester 3.312942e+13
## X1.Octanol 1.809391e+13
## Octanoic.acid..ethyl.ester 7.503234e+13
## Dodecane 6.516700e+12
## Tetradecane 6.834663e+13
## Hexadecane 6.158689e+13
## Hexadecanoic.acid..ethyl.ester 4.510150e+12
## Anisole 2.314498e+14
## Acetophenone 5.762833e+11
## Benzyl.Benzoate 1.172727e+13
## Butanedioic.acid..diethyl.ester 7.852583e+12
## Benzeneacetic.acid..ethyl.ester 9.225659e+13
## Undecane 3.376208e+14
## Decanal 2.188708e+14
## Dodecanal 2.046945e+15
## Hexanal 7.557442e+12
## X2.Hexenal 9.052589e+13
## Decane 4.223017e+11
## Butanoic.acid..3.hexenyl.ester...Z.. 1.125307e+12
## Styrene 1.689171e+13
## X2.6.Octadien.1.ol..3.7.dimethyl....Z.. 1.025900e+16
## X2.6.Octadienal..3.7.dimethyl....Z.. 1.072834e+14
## X2.6.Octadien.1.ol..3.7.dimethyl....E.. 2.029708e+16
## X2.6.Octadienal..3.7.dimethyl. 1.184018e+14
## X2.Propen.1.ol..3.phenyl. 2.316518e+13
## X6.Octen.1.ol..3.7.dimethyl...acetate 8.656904e+14
## X2.6.Octadien.1.ol..3.7.dimethyl...acetate...Z.. 2.030261e+13
## Geranyl.acetate 1.910186e+15
## gamma.Terpinene 5.318343e+11
## Decanoic.acid..ethyl.ester 8.439327e+11
## beta.Phellandrene 4.800984e+12
## PIC.variance.P
## Mass.time 0.1030
## Limonene 0.7010
## X3.Hexen.1.ol...Z.. 0.9140
## X1.Hexanol 0.5745
## beta.Pinene 0.7160
## X3.Hexen.1.ol..acetate...Z.. 0.9150
## trans.beta.Ocimene 0.6470
## Caryophyllene 0.7250
## Humulene 0.6005
## alpha.Farnesene 0.5460
## X3.Carene 0.7305
## Methyl.Benzoate 0.5640
## X.E..beta.Famesene 0.9545
## Germacrene.D 0.9260
## Pentadecane 0.1300
## Heptadecane 0.1790
## alpha.Pinene 0.7260
## Camphene 0.5940
## beta.Myrcene 0.6990
## Tridecane 0.1615
## Tetradecanal 0.1340
## Benzaldehyde 0.2965
## Eucalyptol 0.2150
## X1.Dodecene 0.2470
## Tetradecanoic.acid..ethyl.ester 0.2305
## X1.Octanol 0.4820
## Octanoic.acid..ethyl.ester 0.7595
## Dodecane 0.2655
## Tetradecane 0.0470
## Hexadecane 0.0400
## Hexadecanoic.acid..ethyl.ester 0.2210
## Anisole 0.7750
## Acetophenone 0.4920
## Benzyl.Benzoate 0.4215
## Butanedioic.acid..diethyl.ester 0.4845
## Benzeneacetic.acid..ethyl.ester 0.5100
## Undecane 0.1525
## Decanal 0.1670
## Dodecanal 0.1005
## Hexanal 0.8550
## X2.Hexenal 0.8535
## Decane 0.8780
## Butanoic.acid..3.hexenyl.ester...Z.. 0.8665
## Styrene 0.6790
## X2.6.Octadien.1.ol..3.7.dimethyl....Z.. 0.6920
## X2.6.Octadienal..3.7.dimethyl....Z.. 0.6920
## X2.6.Octadien.1.ol..3.7.dimethyl....E.. 0.6705
## X2.6.Octadienal..3.7.dimethyl. 0.6905
## X2.Propen.1.ol..3.phenyl. 0.6935
## X6.Octen.1.ol..3.7.dimethyl...acetate 0.7380
## X2.6.Octadien.1.ol..3.7.dimethyl...acetate...Z.. 0.6410
## Geranyl.acetate 0.4930
## gamma.Terpinene 0.9445
## Decanoic.acid..ethyl.ester 0.7720
## beta.Phellandrene 0.7655
## PIC.variance.Z
## Mass.time -1.3346675
## Limonene 0.5863442
## X3.Hexen.1.ol...Z.. 1.4104038
## X1.Hexanol 0.3798774
## beta.Pinene 1.1254041
## X3.Hexen.1.ol..acetate...Z.. 1.4248095
## trans.beta.Ocimene 0.5210426
## Caryophyllene 1.0042531
## Humulene 0.2799846
## alpha.Farnesene 0.1610117
## X3.Carene 0.7622148
## Methyl.Benzoate 0.1514969
## X.E..beta.Famesene 1.4207926
## Germacrene.D 1.3865178
## Pentadecane -1.1333024
## Heptadecane -0.9680674
## alpha.Pinene 1.0896686
## Camphene -0.2274729
## beta.Myrcene 0.6700406
## Tridecane -1.0589433
## Tetradecanal -1.0874859
## Benzaldehyde -0.5969023
## Eucalyptol -0.9324200
## X1.Dodecene -0.9040171
## Tetradecanoic.acid..ethyl.ester -0.8922965
## X1.Octanol -0.2151346
## Octanoic.acid..ethyl.ester 1.0872879
## Dodecane -0.8512420
## Tetradecane -1.6268252
## Hexadecane -1.5730135
## Hexadecanoic.acid..ethyl.ester -0.9069957
## Anisole 1.1770463
## Acetophenone -0.4067607
## Benzyl.Benzoate -0.5629336
## Butanedioic.acid..diethyl.ester -0.4033983
## Benzeneacetic.acid..ethyl.ester -0.3415911
## Undecane -1.0550646
## Decanal -1.0809799
## Dodecanal -1.0963241
## Hexanal 1.3336145
## X2.Hexenal 1.3250178
## Decane 1.3757510
## Butanoic.acid..3.hexenyl.ester...Z.. 1.3637025
## Styrene 1.0193620
## X2.6.Octadien.1.ol..3.7.dimethyl....Z.. 1.0521298
## X2.6.Octadienal..3.7.dimethyl....Z.. 1.0577719
## X2.6.Octadien.1.ol..3.7.dimethyl....E.. 1.0011635
## X2.6.Octadienal..3.7.dimethyl. 1.0530120
## X2.Propen.1.ol..3.phenyl. 1.0650846
## X6.Octen.1.ol..3.7.dimethyl...acetate 1.1219431
## X2.6.Octadien.1.ol..3.7.dimethyl...acetate...Z.. 0.4603887
## Geranyl.acetate 0.1954711
## gamma.Terpinene 1.3355970
## Decanoic.acid..ethyl.ester 1.3136440
## beta.Phellandrene 1.2812382
We can now calculate Blombergs K with the entire dataset as a whole. I thikn multivariate comparative methods are still in their infense and a lot of work is being done figure out how to deal with multivarite data. There are two libraries that have this implementation, Phylocurve and Geomorph.
PhyloCurve Implementation
model <- evo.model(trimedTree, avgScentCompounds)
K.mult(model, nsim = 1000, plot = TRUE)
##
## Bootstrapping under null model.
##
## **********Simulation results**********
## Test statistic (K) 0.2612838
## Critical test statistic 0.6072138
## Estimated Power 0.7240000
##
## P-value: 0.96
Figure Interpretation: Black density plot is null distribution of K values from 1000 simulations of Brownian motion under a star phylogeny. Blue is a distribution of K values from 1000 simulations on the true phylogeny. Black dotted line is observe K value from multiveriate Bloomberg’s K on observed data. This value falls well within the null distribution of simulation, indicating that there is no phylogenetic signal.
Geomorph Implimentation
zzz<-physignal(avgScentCompounds,trimedTree)
##
|
| | 0%
|
| | 1%
|
|= | 1%
|
|= | 2%
|
|== | 2%
|
|== | 3%
|
|== | 4%
|
|=== | 4%
|
|=== | 5%
|
|==== | 5%
|
|==== | 6%
|
|==== | 7%
|
|===== | 7%
|
|===== | 8%
|
|====== | 8%
|
|====== | 9%
|
|====== | 10%
|
|======= | 10%
|
|======= | 11%
|
|======= | 12%
|
|======== | 12%
|
|======== | 13%
|
|========= | 13%
|
|========= | 14%
|
|========= | 15%
|
|========== | 15%
|
|========== | 16%
|
|=========== | 16%
|
|=========== | 17%
|
|=========== | 18%
|
|============ | 18%
|
|============ | 19%
|
|============= | 19%
|
|============= | 20%
|
|============= | 21%
|
|============== | 21%
|
|============== | 22%
|
|=============== | 22%
|
|=============== | 23%
|
|=============== | 24%
|
|================ | 24%
|
|================ | 25%
|
|================= | 25%
|
|================= | 26%
|
|================= | 27%
|
|================== | 27%
|
|================== | 28%
|
|=================== | 28%
|
|=================== | 29%
|
|=================== | 30%
|
|==================== | 30%
|
|==================== | 31%
|
|==================== | 32%
|
|===================== | 32%
|
|===================== | 33%
|
|====================== | 33%
|
|====================== | 34%
|
|====================== | 35%
|
|======================= | 35%
|
|======================= | 36%
|
|======================== | 36%
|
|======================== | 37%
|
|======================== | 38%
|
|========================= | 38%
|
|========================= | 39%
|
|========================== | 39%
|
|========================== | 40%
|
|========================== | 41%
|
|=========================== | 41%
|
|=========================== | 42%
|
|============================ | 42%
|
|============================ | 43%
|
|============================ | 44%
|
|============================= | 44%
|
|============================= | 45%
|
|============================== | 45%
|
|============================== | 46%
|
|============================== | 47%
|
|=============================== | 47%
|
|=============================== | 48%
|
|================================ | 48%
|
|================================ | 49%
|
|================================ | 50%
|
|================================= | 50%
|
|================================= | 51%
|
|================================= | 52%
|
|================================== | 52%
|
|================================== | 53%
|
|=================================== | 53%
|
|=================================== | 54%
|
|=================================== | 55%
|
|==================================== | 55%
|
|==================================== | 56%
|
|===================================== | 56%
|
|===================================== | 57%
|
|===================================== | 58%
|
|====================================== | 58%
|
|====================================== | 59%
|
|======================================= | 59%
|
|======================================= | 60%
|
|======================================= | 61%
|
|======================================== | 61%
|
|======================================== | 62%
|
|========================================= | 62%
|
|========================================= | 63%
|
|========================================= | 64%
|
|========================================== | 64%
|
|========================================== | 65%
|
|=========================================== | 65%
|
|=========================================== | 66%
|
|=========================================== | 67%
|
|============================================ | 67%
|
|============================================ | 68%
|
|============================================= | 68%
|
|============================================= | 69%
|
|============================================= | 70%
|
|============================================== | 70%
|
|============================================== | 71%
|
|============================================== | 72%
|
|=============================================== | 72%
|
|=============================================== | 73%
|
|================================================ | 73%
|
|================================================ | 74%
|
|================================================ | 75%
|
|================================================= | 75%
|
|================================================= | 76%
|
|================================================== | 76%
|
|================================================== | 77%
|
|================================================== | 78%
|
|=================================================== | 78%
|
|=================================================== | 79%
|
|==================================================== | 79%
|
|==================================================== | 80%
|
|==================================================== | 81%
|
|===================================================== | 81%
|
|===================================================== | 82%
|
|====================================================== | 82%
|
|====================================================== | 83%
|
|====================================================== | 84%
|
|======================================================= | 84%
|
|======================================================= | 85%
|
|======================================================== | 85%
|
|======================================================== | 86%
|
|======================================================== | 87%
|
|========================================================= | 87%
|
|========================================================= | 88%
|
|========================================================== | 88%
|
|========================================================== | 89%
|
|========================================================== | 90%
|
|=========================================================== | 90%
|
|=========================================================== | 91%
|
|=========================================================== | 92%
|
|============================================================ | 92%
|
|============================================================ | 93%
|
|============================================================= | 93%
|
|============================================================= | 94%
|
|============================================================= | 95%
|
|============================================================== | 95%
|
|============================================================== | 96%
|
|=============================================================== | 96%
|
|=============================================================== | 97%
|
|=============================================================== | 98%
|
|================================================================ | 98%
|
|================================================================ | 99%
|
|=================================================================| 99%
|
|=================================================================| 100%
Test for Phylogenetic Signal
In addition to the VOC compounds we had an additional data source.
Prune Tree down to 8 species with EAG data
keepSpecies <- EAG$Species_Name_Full
trimedTree<-drop.tip(tree,tree$tip.label[-match(keepSpecies, tree$tip.label)])
plot(trimedTree)
Data Wrangle EAG Response
continuousResponse <- as.matrix(data.frame(row.names = EAG$Species_Name_Full,
EAG$EAG))[,1]
sigResponses <- as.matrix(data.frame(row.names = EAG$Species_Name_Full,
EAG$EAG_Sig))[,1]
EAGBloombergsK <- as.matrix(continuousResponse)
multiPhylosignal(EAGBloombergsK, tree)
## K PIC.variance.obs PIC.variance.rnd.mean PIC.variance.P
## 1 0.2366938 12.0132 6.715752 0.999
## PIC.variance.Z
## 1 2.900658
Ancestrl State Reconstruction
g <- contMap(trimedTree, continuousResponse, plot = TRUE, type="phylogram")
# Testing between ER and ARD
results.anc <- data.frame( model=c("ER","ARD"),
lnL=numeric(2),
AICc=numeric(2),
params=numeric(2))
ER_fit <- fitDiscrete(trimedTree, sigResponses, model="ER")
results.anc[1,-1]<- c(lnL=ER_fit$opt$lnL,AICc=ER_fit$opt$aicc,ER_fit$opt$k)
ARD_fit <- fitDiscrete(trimedTree, sigResponses, model="ARD")
results.anc[2,-1]<- c(lnL=ARD_fit$opt$lnL,AICc=ARD_fit$opt$aicc,ARD_fit$opt$k)
results.anc <- results.anc[order(results.anc$AICc),]
results.anc
## model lnL AICc params
## 1 ER -5.545177 13.75702 1
## 2 ARD -5.545177 17.49035 2
# Plotting Joint Marginal Estimation of ER
z <- as.factor(sigResponses)
ERreconstruction <- ace(z, trimedTree, type="discrete", model="ER")
plotTree(trimedTree, setEnv = TRUE, offset = 0.5)
tiplabels(pie = to.matrix(z, sort(unique(z))),
piecol = c("#F8766D", "#00BFC4"),
cex = 0.4)
nodelabels( node=1:trimedTree$Nnode+Ntip(trimedTree),
pie = ERreconstruction$lik.anc,
cex=0.6,
piecol = c("#F8766D", "#00BFC4"))
nodelabels(
pie = ERreconstruction$lik.anc,
piecol = c("#F8766D", "#00BFC4"),
cex = 0.6)
legend("topleft",
legend=c("Significant EAG", "Non-Significant EAG"),
pch=20,
col=c("#F8766D", "#00BFC4"),
bty="n",
text.col="gray32",
cex=0.8,
pt.cex=1.5)
set.seed(1234)
cols <- setNames(c("#1b9e77","#7570b3","#d95f02"), sort(unique(sigResponses)))
chartrees <- make.simmap(trimedTree, sigResponses, model='ER', nsim = 200)
## make.simmap is sampling character histories conditioned on the transition matrix
##
## Q =
## 1 2
## 1 -1057.981 1057.981
## 2 1057.981 -1057.981
## (estimated using likelihood);
## and (mean) root node prior probabilities
## pi =
## 1 2
## 0.5 0.5
(res_simmap <- describe.simmap(chartrees, plot = FALSE))
## 200 trees with a mapped discrete character with states:
## 1, 2
##
## trees have 122.46 changes between states on average
##
## changes are of the following types:
## 1,2 2,1
## x->y 61.18 61.28
##
## mean total time spent in each state is:
## 1 2 total
## raw 0.05728896 0.0570632 0.1143522
## prop 0.50098713 0.4990129 1.0000000
plot( trimedTree,type="p",
FALSE,label.offset=0.6,
cex=0.6,
no.margin=TRUE,
edge.color="gray32",
tip.color="gray32")
tiplabels( pch=21,bg=cols[as.numeric(sigResponses)],
col="gray32",
cex=1,
adj=0.6)
nodelabels( pie=res_simmap$ace,
piecol=cols,
cex=0.5,
col="gray32")
#legend("bottomleft",
# legend=levels(sigResponses),
# pch=20,
# col="gray32",
# bty="n",
# text.col="gray32",
# cex=0.8,
# pt.cex=1.5)
#plotSimmap(chartrees, cols, pts = FALSE, lwd = 3, fsize=0.6)
#add.simmap.legend(colors = cols, vertical = FALSE, prompt = FALSE, x = 0, y = 1)
References Jones et al. 2015